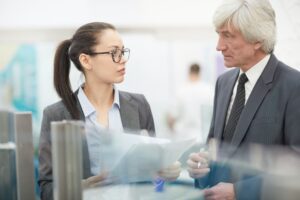
The conglomerate term of Data Analytics refers to several applications which perform activities such as:
Denizon leverages advanced computer skills, statistics, and mathematics to extract actionable insights from your data. Our data analytics services enable predictive modeling and provide valuable knowledge to fuel your future predictive analytics initiatives.
Data analytics processes do not just analyse data. Before the actual analysis, the data has to be readied to apply the analytics model. The process of data analytics includes the following:
Depending on the business needs, data analytics are of the following four types:
Descriptive analytics answers the question of what happened. It analyses the available data to learn about past events. It juggles raw data from multiple sources to give insights into the past. Descriptive analytics however only signals that something has happened, without dwelling into the reason why.
Diagnostic analytics answers the question of why something happened. After carrying out descriptive analytics, diagnostic analytics allows a business to drill down issues, find out dependencies, and identify patterns. Diagnostic analytics essentially gives in-depth insights into a particular problem to measure risks.
Prescriptive Analytics prescribes what action can be taken to eliminate a future problem or to take advantage of a promising trend. The prescriptive analysis uses sophisticated tools and technologies like machine learning, business rules, and algorithms. It helps organisations identify opportunities for repeated activities.
As the name suggests, predictive analytics tells what is likely to happen. It uses the combination of descriptive and diagnostic analytics to detect tendencies and predict future trends based on the analysis. It allows businesses to carry out activities such as triggering targeted marketing activities, weighing in the risks of network failures, and executing cash flow analysis.
Data analytics offers knowledge discovery and prediction capabilities, thus helping businesses understand the current state of the business process. Data analytics helps create a solid foundation to predict outcomes and create products that meet future needs. Hence, data analytics plays a major role in shaping the pathway for product development.
Data analytics helps businesses become proactive by anticipating the needs of their customers. When customers share their data with businesses, they expect the business to know them and provide a seamless experience by anticipating their needs. Hence, data analytics helps provide this experience and ultimately optimise the customer experience.
Data collation combined with analytics helps businesses anticipate market demands and personalise marketing campaigns. Analytics helps businesses determine the exact marketing segment that a customer base will respond to best. Ultimately, personalising and targeting content allows businesses to save money on convincing customers to make purchases.
Data analytics ultimately leads to increased operational efficiency. It helps companies and businesses identify potential opportunities, streamline, and maximise profits. It allows companies to identify the operations that yield the best results and the operations that don’t. It also helps determine the business processes that need to be improved and the error-free processes.
Efficient data analytics delivers fraud prevention and organisational security capabilities. Data management, efficient and transparent reporting of fraud incidents, and data analytics tools that offer intrusion detection capabilities make it possible for businesses to mitigate risk and fraud.
Predictive analysis is used in the policing/security domain to predict surges in crimes depending on the time of the year or the time of the day.
Predictive analysis is used in the policing/security domain to predict surges in crimes depending on the time of the year or the time of the day.
One of the initial applications of data analytics is the ability to detect fraud and risk. Data analytics helps detect patterns and use those patterns to predict the presence of fraud and risky activities. This fraud and risk detection can be done both in the financial sector as well as the network domain sector. Continuous data collection and analytics helps detect and keep away security issues.
Risk management is another application of data analytics. Data analytics helps organisations assess data, and evaluate risks based on historical data and failures.
Data analytics has also paved its way into affecting delivery logistics. Logistic companies can use the results from data analytics to find suitable shipping routes, best delivery times, and the most suitable means of transportation.
Internet provision is another application of data analytics. Providing fast internet services in the right place at the right time requires the analysis and prediction of data. Shifting the bandwidth at the right time and location is crucial, and this can only be achieved by using the results obtained from data analytics.
Spending the taxpayers budget or a company budget in the right place at the right time is crucial for success. Data analytics applications help determine the exact areas where the money should be spent and the exact time and condition which reaps the best benefits.
Regular customer surveys and gathering information is another crucial application of data analytics. This data helps to understand the business services that are being used more, and the services which need improvement. Customer interactions are another way of understanding and determining what the future of the business must look like.
Data analytics helps travellers optimise their travelling experiences by knowing exactly where to go, what to buy, and where to stay based on the data collected from previous travellers. A traveller’s experiences, preferences, and desires can be used to deliver personalised recommendations, thus leading to a richer, better experience.
Search engines like Google, Bing, Yahoo, AOL, Ask, and DuckDuckgo use data analytics to deliver the best results for any search query put into the search bar. Without data sciences and analytics, it is impossible for search engines to find search results in a split second.
Another area where data analytics comes to play is digital advertisement. Data algorithms control all the digital advertisement details, and hence digital adverts get more CTR (Click-through Rate) than the conventional advertisement methods. The past behaviour of users helps create better-structured advertisements that reap proper benefits.
The amount of data being collected by every organisation is extensive. Every organisation receives information about every incident and interaction leaving analysts boggling about a huge amount of interlocking data sets.
The main challenge in collecting data is to deploy a manual process to do so. An automated system to collect data helps to utilise the time spent in collecting data actually to act on it instead.
The amount of data being collected by every organisation is extensive. Every organisation receives information about every incident and interaction leaving analysts boggling about a huge amount of interlocking data sets.
The main challenge in collecting data is to deploy a manual process to do so. An automated system to collect data helps to utilise the time spent in collecting data actually to act on it instead.
Data needs to be visually represented in a proper graph, chart, or tabular format for it to be impactful. It is often frustrating to pull information from multiple areas and feed it into a visualisation tool.
Every source of data is spread across different, disjointed sources and are hosted in different systems. This is always not known; hence data collection can at times be incompletely collected and inaccurately analysed. Hence, to cater to this challenge, it is always good to create a centralised system to access data and analyse it from a centralised location.
Every source of data is spread across different, disjointed sources and are hosted in different systems. This is always not known; hence data collection can at times be incompletely collected and inaccurately analysed. Hence, to cater to this challenge, it is always good to create a centralised system to access data and analyse it from a centralised location.
Lack of talent and skills is another major challenge for data analytics. For companies without risk departments and human analytics resources, data analytics can be a cumbersome deal. Shortage of skills can be dealt with in two ways; by hiring a skilled resource or by outsourcing data analytics. While the first solution ensures that the organisation has skilled manpower in their hand, the second solution will ensure that even a layman can utilise the data analytics system regardless of their data analytics skills.
Outsourcing data analytics to us brings numerous benefits for small and mid-level organizations. We streamline the management of your data, ensuring it is transformed into valuable information. Our expertise in data analytics allows you to gain insights, improve efficiency, and achieve cost savings.
Our team of highly skilled and experienced data professionals excels in the art of data management. They meticulously collect, analyze, and manipulate data from various sources to unveil valuable insights and deliver strategic solutions. With their expertise, your organization will gain a powerful advantage, enabling you to make informed decisions, drive innovation, and outperform the competition.
Outsourcing data analytics with us unlocks your organization’s full potential by freeing up your internal resources and allowing them to concentrate on core business tasks. Our specialized expertise in data analytics ensures accurate and insightful predictions, empowering you to make informed decisions that drive growth and maximize your profitability. With our services, you can harness the power of data to unlock new opportunities and gain a competitive edge in the market.
Outsourcing data analytics with us is a cost-effective solution for small and mid-sized organizations. By leveraging our expertise, you eliminate the need to hire and maintain an in-house team of specialized software engineers, data analysis experts, project managers, and database specialists. This results in significant cost savings, allowing you to allocate your resources more efficiently and focus on your core business functions. With our affordable and scalable data analytics services, you can access high-quality insights without straining your budget.
At Denizon, we employ advanced and specialized tools for data analysis, organization, and visualization. These tools enable us to tackle complex challenges faced by organizations, making the process of problem identification and solution formulation efficient and effective. With our expertise as data analytics experts and the power of these tools, we ensure that your day-to-day operations run smoothly and seamlessly. By harnessing the capabilities of these tools, we provide valuable insights and actionable recommendations that can drive informed decision-making and optimize your business processes.
We specialize in enhancing your data management practices across various platforms on a daily, monthly, and yearly basis. Our goal is to help you streamline and systemize your data, ensuring that the right information is readily available at the right time and in the right place. With our expertise, we assist in organizing and structuring your data in a manner that enables easy accessibility and retrieval whenever you need it. By implementing robust data management systems and processes, we ensure that you have a reliable and efficient data infrastructure that supports your business operations and decision-making processes.
Outsourcing your data analytics needs to us not only provides you with expertise but also helps you save valuable time. Our experienced team knows the ins and outs of data analytics, enabling us to efficiently handle tasks and projects within tight timelines. With our streamlined processes and optimized workflows, we can accomplish a significant amount of work in a shorter duration. By leveraging our expertise and efficient time management, you can focus on other core aspects of your business while trusting us to deliver accurate and actionable insights from your data.
Often organisations focus less on how to secure their crucial data and focus more on how to analyse it and obtain crucial results. We at Denizon make sure that your data is protected the confidentiality of your data is maintained at all times.
Get advice on the benefits of Data Analytics in terms of Operational Efficiency, Product Development, Risk Mitigation and Fraud.